Introduction
The field of drug discovery is undergoing a remarkable transformation, fueled by advances in both lab technology and the software used to parse, store, analyze, and transform data. Target identification and validation are the foundational steps that kick-start the drug discovery research cycle and historically require an intensive effort. This could include high-throughput screening in lab and data processing.
By employing metrics-driven strategies, scientists are pushing the boundaries of drug discovery and finding novel, more efficient ways to find drug targets. In this article, we dive into the fascinating world of target identification and validation, exploring their significance, recent advancements, and the impact they have on the future of drug discovery.
What is Target Identification?
A drug target can be defined as the specific biological entity that a drug aims to interact with. Typically, when the biological process behind a disease is relatively well understood, the target is a biological entity involved in causing the disease. Drug targets can include DNA, RNA, proteins, enzymes, receptors, or other molecules that play a crucial role in the development or progression of a particular condition.
By targeting specific molecules, drugs can intervene in the underlying mechanisms of a disease. This could show up in two ways - 1) blocking harmful processes or 2) promoting beneficial ones. The identification and validation of drug targets are crucial steps in the development of new medications and therapies to treat diseases.
A popular example that everyone can relate to—Aspirin. Aspirin is a commonly used medication that belongs to the class of non-steroidal anti-inflammatory drugs (NSAIDs). It is widely known for its analgesic (pain-relieving), anti-inflammatory, and antipyretic (fever-reducing) properties.
The drug target of Aspirin is the Cyclooxygenase-1 (COX-1) enzyme. COX-1 is an enzyme that plays a role in the production of certain prostaglandins, which are chemical messengers involved in pain, inflammation, and regulation of various physiological processes. Aspirin works by inhibiting the activity of the COX-1 enzyme, thereby reducing the production of prostaglandins.
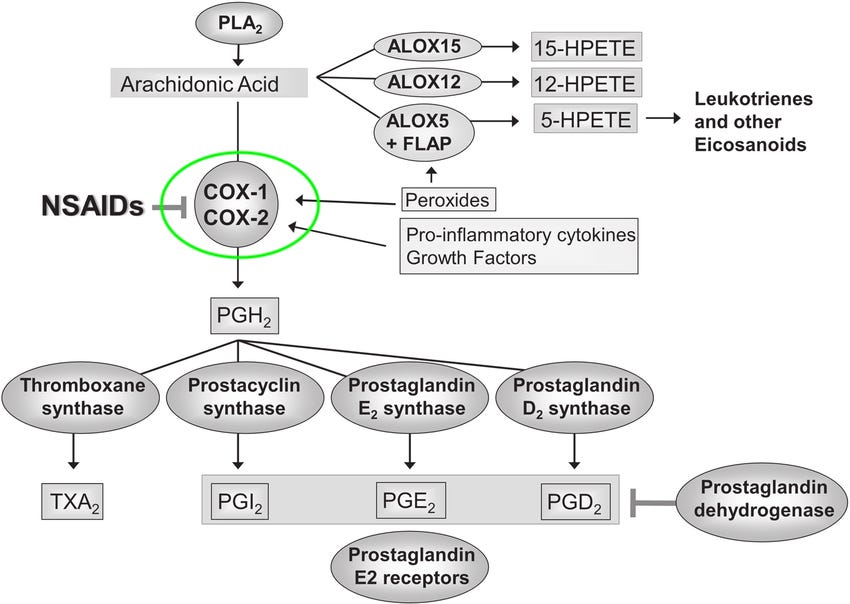
What are the requirements for a good drug target?
Target should be present
Determining what qualifies as a good target is a complex matter. One essential aspect is that the target has to be actively involved in the disease process. This may not be the case in some instances where a disease can be caused due to the absence of a gene or protein. In other words, for a drug to be effective in treating a disease, it needs to act upon a target that is present within the body. If the target is absent or not present in sufficient quantities, the drug may not achieve the desired therapeutic outcome. In short, the target must be an active agent in causation of the disease and this is a fundamental prerequisite for the effectiveness of a drug. A good example of this is the treatment of the condition called cystic fibrosis, where non-sense mutations of the CFTR gene can cause the disease. However, since CFTR is not present, it becomes an unsuitable focus for therapeutic intervention. In simpler terms, even if discovery research identifies a biochemically suitable target, attempting to manipulate something that is not abundantly available, like the CFTR protein in this case, is not feasible.
In the case of CFTR, scientists found ways of targeting specific deletions on the CFTR protein (where the deletions behaved more like missense mutations) and for generally upregulating the number of chloride transporters (targeting upstream production).
Target should be linked with causation of the disease
In addition to being present, a protein should be causative to a disease phenotype. Establishing causation is very difficult in biological systems because there are so many feedback loops meant to adjust for reduced or increased activity of any given biomolecule.
The most famous case of a non-causative disease protein is B-amyloid in Alzheimer’s disease. We know it is present, but after decades of research we’ve still been unable to determine if it’s causative. Judging by the mixed results produced by drugs targeting mutated B-amyloid, it appears to be the case that it’s not causative of Alzheimer’s symptoms.
How do drug discovery scientists validate targets?
Once you’ve established that the target is causative, then the question becomes: can you hit it? When it comes to hunting down those elusive drug targets, their location can make all the difference. Think of it this way: the easier a target is to reach, the more likely it is to be successfully hit. So, where do these elusive targets like to hang out?
The targets that are easiest to hit are those that are exposed on the cellular membrane - targets that are inside the cell mean that certain classes of therapeutics won’t be able to reach them there. Targets that are inside the nucleus are even harder to hit (notoriously - antibody therapeutics usually can’t get here). Many oncogenes, which are in the nucleus) are related to DNA repair and proliferation, and that makes cancer therapeutics particularly difficult to develop. But the process is not as simple as finding a hittable target. Once a target candidate has been identified the next key checks necessary are:
Hitting a target shouldn’t create other downstream issues
If a hittable target is critical for some other process there might be unintended negative impacts of using it for therapeutic intervention. For examples, signal-transduction pathways, which regulate various biological functions, often face a challenge as potential drug targets. Although targeting these pathways could improve the disease, it would also impact multiple other systems, rendering it impractical as a usable target.
The candidate drug should not hit other things
This criteria is simply asking the question - does the drug hit “non targets”? This is most commonly seen for small molecule drugs that can “fit into the pocket” of multiple enzymes, and for antibodies that on, on first screen, hit the target but also hit common epitopes on others. They make great therapeutics and have exactly the effect on the target that you expect…but unfortunately they also hit a bunch of other things that may be necessary for normal processes. This in turn can result in drug toxicity and safety concerns.
Both of these situations fall under the category of 'off-target' effects. It means that either hitting the desired target produces undesired effects, or the therapeutic intervention affects additional targets along with the intended one.
How is target identification done today?
In the traditional approach of target identification, researchers typically focus on individuals with a specific disease and search for proteins that play a role in causing the observed symptoms. In heart disease, if cholesterol is believed to be a major contributor to its complications, scientists would examine the pathway involved and identify HMG-CoA reductase as a crucial enzyme responsible for cholesterol synthesis in the liver. By developing a drug that inhibits this enzyme, the production of cholesterol can be effectively prevented.
For other diseases, rather than an up-regulation of an existing process, it’s a mutation in a gene that is the root cause of disease. One of the most common examples is seen in cancer, where specific oncogenes undergo mutations in the diseased state. Let's take non-small-cell lung cancer. In a subset of such cancers, a particular mutation in the EGFR gene leads to the production of a distorted protein that promotes the uncontrolled growth of cancer cells. This misshapen protein becomes an ideal target for therapeutic interventions. Over time, multiple generations of drugs have been developed to specifically target EGFR, with the most recent one being Osimertinib. These drugs aim to counteract the effects of the mutated EGFR protein and hinder the progression of the disease.
The efficacy of these treatments begs the question - how do you identify misshapen or up-regulated proteins in the disease state? To identify misshapen or up-regulated proteins in the disease state, researchers start with a model for the disease. In the case of cancer, where cells undergo mutation and “become” proliferative, the disease model can be built using genomic data gathered from the tumors of patients. By examining genomic mutations or biomarkers that consistently appear in predictable patterns in these patients, they can pinpoint potential abnormalities in protein structure or expression associated with the disease.
In other diseases, cells can be assayed in vitro to understand the model - for example, cardiac cells in a dish are often a useful way to understand cardiac arrhythmias.
Data landscape and novel computational techniques
While most biotech organizations continue to identify targets (and potential candidates) using traditional techniques, the advent of data ecosystems around target selection mean that they can do their analysis much faster via software and automation. For example, many scientists now use digital pathway analysis alongside the traditional method, literature review, to identify potential targets. It’s easier to see what the potential off-target effects might be for a given target that way - homology can be determined for similar proteins, and it’s clear what other parts are critical downstream. With the advent of the open source protein prediction software, such as AlphaFold, scientists should be able to run virtual screens more efficiently than they could before. Theoretically, this would help them identify inaccessible binding pockets, conformational variability in the target, and further define the binding site beforehand. That said, Alphafold’s impact on drug-target interactions is unclear [5]. Companies like Recursion, Insitro, BenevolentAI and Atomwise are already using AI/ML led predictive technologies to accelerate the process of target identification.
Scientists can learn from historical data on drug candidates and adjust their expectations when exploring new ones. For instance, if they find that a specific target caused liver damage in a previous drug, they know that hitting the same target with a different candidate carries a similar risk. With the total number of genes in our bodies being relatively small, in the tens of thousands, researchers are gradually gaining a comprehensive understanding of each protein and its downstream effects. As a result, the utilization of prior knowledge becomes increasingly crucial in drug development. Although the work done by academic labs is available via peer-reviewed journals, quite a lot of knowledge on this topic remains siloed within research organizations at biotech and biopharma companies. When utilized to its full potential, this knowledge can enable scientists to make more informed decisions and minimize potential risks associated with off-target effects, ultimately bringing us closer to more effective and safer therapies.
References
[1] Resler, Alexa & Makar, Karen & Heath, Laura & Whitton, John & Potter, John & Poole, Elizabeth & Habermann, Nina & Scherer, Dominique & Duggan, David & Wang, Hansong & Lindor, Noralane & Passarelli, Michael & Baron, John & Newcomb, Polly & Marchand, Loic & Ulrich, Cornelia. (2014). Genetic variation in prostaglandin synthesis and related pathways, NSAID use and colorectal cancer risk in the Colon Cancer Family Registry. Carcinogenesis. 35. 10.1093/carcin/bgu119.
[2] Garcia-Fernandez-Bravo et al. Undertreatment or Overtreatment With Statins: Where Are We? Front. Cardiovasc. Med., 29 April 2022 Sec. Lipids in Cardiovascular Disease Volume 9 - 2022 | https://doi.org/10.3389/fcvm.2022.808712
[3] Santarpia, Mariacarmela & Liguori, Alessia & Karachaliou, Niki & Cao, Maria & Daffinà, Maria & D'Aveni, Alessandro & Marabello, Grazia & Altavilla, Giuseppe & Rosell, Rafael. (2017). Osimertinib in the treatment of non-small-cell lung cancer: Design, development and place in therapy. Lung Cancer: Targets and Therapy. Volume 8. 109-125. 10.2147/LCTT.S119644.
[4] Tokheim C, Karchin R. CHASMplus Reveals the Scope of Somatic Missense Mutations Driving Human Cancers. Cell Syst. 2019 Jul 24;9(1):9-23.e8. doi: 10.1016/j.cels.2019.05.005. Epub 2019 Jun 12. PMID: 31202631; PMCID: PMC6857794.
[5] Scardino V, Di Filippo JI, Cavasotto CN. How good are AlphaFold models for docking-based virtual screening? iScience. 2022 Dec 30;26(1):105920. doi: 10.1016/j.isci.2022.105920. PMID: 36686396; PMCID: PMC9852548.
[6] Felix Wong, Aarti Krishnan, Erica Zheng, Hannes Stärk, Abigail Manson, Ashlee M. Earl, Tommi Jaakkola, and James Collins. Benchmarking AlphaFold-enabled molecular docking. Mol Syst Biol. (2022) 18: e11081.
Sanjay Saraf is a PM at Benchling, where he helps biotech companies solve data analysis, automation, and preclinical research problems. He is now focused on Studies, a product which enables scientists to capture accurate, intelligent, and connected in vivo study data. Prior to Benchling, Sanjay led healthcare and biology partnerships at Palantir Technologies, focusing on pharma, government, and clinical organizations.
Vega Shah is a PMM at Benchling. She leads the research-specific applications of Benchling in biotech and pharma. Previously she was a PM at Dotmatics for ELN and integrations. Before her industry career she was a scientific researcher at Lawrence Berkeley National Lab, UW, and UC Berkeley.